What is Closenet?
Closenet is a specialized tool in the field of natural language processing (NLP) that focuses on extracting relevant information and relationships from large bodies of text. It is a type of information extraction system that leverages machine learning and natural language understanding techniques to identify and classify entities, concepts, and their interconnections within a given text.
Closenet is particularly valuable for tasks such as named entity recognition (NER), relation extraction, and event extraction. These capabilities make it a crucial component in various NLP applications, including question answering systems, text summarization, and machine translation. By harnessing Closenet's capabilities, researchers and practitioners can unlock insights from unstructured text data, leading to improved performance and accuracy in NLP tasks.
To delve deeper into the significance and applications of Closenet, let's explore its historical context, evolution, and how it has revolutionized the field of NLP.
In recent years, Closenet has gained prominence as a powerful tool for information extraction. Its ability to handle complex text, identify intricate relationships, and extract meaningful insights has made it a cornerstone of modern NLP pipelines. As we continue to explore the frontiers of NLP, Closenet is poised to play an increasingly vital role in shaping the future of information extraction and text analysis.
Closenet
Closenet is a powerful tool in the field of natural language processing (NLP) that specializes in extracting relevant information and relationships from large bodies of text. Its capabilities include named entity recognition (NER), relation extraction, and event extraction. Here are six key aspects that highlight the significance of Closenet:
- Information Extraction: Closenet excels in extracting structured data from unstructured text, making it valuable for tasks like question answering and text summarization.
- Machine Learning Foundation: It leverages machine learning algorithms to identify and classify entities, concepts, and their relationships, improving its accuracy over time.
- Natural Language Understanding: Closenet's ability to understand the context and meaning of text enables it to extract insights beyond just keywords.
- Entity Recognition: It can identify and categorize named entities such as people, organizations, locations, and temporal expressions.
- Relationship Extraction: Closenet detects and classifies the relationships between entities, providing a deeper understanding of the text's structure.
- Event Extraction: It extracts events and their attributes, including participants, time, and location, from textual data.
These aspects collectively make Closenet a crucial component in NLP applications. Its ability to unlock insights from unstructured text data has revolutionized the field of information extraction. Closenet continues to empower researchers and practitioners in various domains, including information retrieval, text mining, and machine translation.
Information Extraction
Information extraction is a fundamental aspect of Closenet's capabilities. Its ability to extract structured data from unstructured text sets it apart as a valuable tool for various NLP tasks, particularly question answering and text summarization.
In question answering systems, Closenet plays a crucial role in identifying and extracting relevant information from large text corpora. By accurately extracting entities, relationships, and events, Closenet enables these systems to provide precise and comprehensive answers to complex questions posed by users.
Similarly, in text summarization, Closenet's ability to extract key information and organize it in a coherent manner makes it a valuable component. It helps identify the main points, supporting details, and relationships within a text, enabling the creation of concise and informative summaries.
The practical significance of this understanding lies in the improved accuracy and efficiency of NLP applications. By leveraging Closenet's information extraction capabilities, researchers and practitioners can develop more effective question answering systems, text summarization tools, and other NLP-powered applications.
Machine Learning Foundation
The machine learning foundation of Closenet plays a pivotal role in its ability to identify and classify entities, concepts, and their relationships within text. This foundation provides Closenet with several key advantages:
- Enhanced Accuracy: Machine learning algorithms enable Closenet to learn from large datasets, continually improving its accuracy in identifying and classifying information.
- Adaptability: Closenet can adapt to new domains and languages, as machine learning algorithms allow it to learn from new data and adjust its models accordingly.
- Automation: Machine learning automates the process of feature extraction and model building, reducing the need for manual intervention and streamlining the information extraction process.
- Scalability: Closenet's machine learning foundation enables it to handle large volumes of text data efficiently, making it suitable for big data applications.
These advantages collectively contribute to Closenet's effectiveness in extracting structured information from unstructured text, making it a valuable tool for various NLP tasks.
Natural Language Understanding
Natural language understanding (NLU) is a crucial aspect of Closenet's capabilities, as it allows the tool to comprehend the context and meaning of text, leading to more accurate and insightful information extraction. NLU enables Closenet to go beyond merely identifying keywords and phrases; it can grasp the underlying relationships and concepts within the text.
This ability is particularly valuable in tasks such as sentiment analysis, where Closenet can determine the sentiment expressed in a piece of text by understanding the context and tone. Additionally, NLU enables Closenet to perform more complex tasks such as question answering, where it needs to comprehend the intent and context of a question to provide a relevant and informative answer.
The practical significance of Closenet's NLU capabilities is evident in its applications across various domains. For instance, in the healthcare industry, Closenet is used to analyze patient records and extract relevant information, such as symptoms, diagnoses, and treatment plans. By understanding the context and meaning of the medical text, Closenet can assist healthcare professionals in making more informed decisions and improving patient care.
In conclusion, Closenet's NLU capabilities are fundamental to its effectiveness in extracting insights from unstructured text. By understanding the context and meaning of text, Closenet can provide more accurate and comprehensive information, leading to improved performance in various NLP tasks and real-world applications.
Entity Recognition
Entity recognition is a fundamental component of Closenet's capabilities, enabling it to identify and categorize named entities within text. These named entities can include people, organizations, locations, and temporal expressions, providing valuable information for various NLP tasks.
The accurate recognition of named entities is crucial for many NLP applications. For instance, in information extraction systems, correctly identifying named entities allows for the extraction of structured data, facilitating tasks such as populating knowledge graphs and creating structured databases from unstructured text. Additionally, in question answering systems, recognizing named entities enables the system to identify and extract relevant information from text to answer user queries.
Closenet's entity recognition capabilities are particularly valuable in domains such as finance and healthcare. In the financial domain, Closenet can be used to identify and categorize financial entities such as companies, stocks, and financial instruments, enabling the extraction of financial data from news articles and reports. In healthcare, Closenet can identify and categorize medical entities such as diseases, drugs, and medical procedures, facilitating the extraction of relevant information from medical records and research papers.
The practical significance of Closenet's entity recognition capabilities extends to various real-world applications. For instance, in the legal domain, Closenet can be used to analyze legal documents and identify key entities such as parties involved, legal terms, and precedents, aiding in legal research and decision-making. In the media industry, Closenet can be used to analyze news articles and identify key entities and their relationships, providing insights into public opinion and trends.
In summary, Closenet's entity recognition capabilities play a vital role in extracting structured information from unstructured text. The ability to identify and categorize named entities enables a wide range of NLP applications, providing valuable insights and facilitating tasks across diverse domains.
Relationship Extraction
Relationship extraction is a crucial aspect of Closenet's capabilities, enabling it to identify and classify the relationships between entities within text, providing a deeper understanding of the text's structure and the relationships between the entities it contains.
- Identifying Relationships: Closenet can identify various types of relationships between entities, including semantic relationships (e.g., Person-Organization, Location-Event), syntactic relationships (e.g., subject-object, verb-object), and temporal relationships (e.g., before, after, during).
- Real-World Examples: Relationship extraction finds applications in various domains. In the legal domain, it can be used to identify relationships between legal entities and concepts within legal documents. In the healthcare domain, it can be used to extract relationships between medical entities, such as diseases and treatments, from medical records.
- Enhancing NLP Applications: The ability to extract relationships between entities enhances the performance of NLP applications. In question answering systems, relationship extraction enables the system to provide more comprehensive and informative answers by understanding the relationships between the entities in the question and the text.
- Facilitating Data Analysis: Relationship extraction also plays a vital role in data analysis tasks. By identifying relationships between entities in unstructured text, Closenet enables the extraction of structured data, which can be further analyzed to derive insights and make informed decisions.
In conclusion, Closenet's relationship extraction capabilities provide a deeper understanding of the text's structure and the relationships between entities within the text. This capability enhances the performance of NLP applications, facilitates data analysis tasks, and finds applications in various domains, making Closenet an essential tool for information extraction and text analysis.
Event Extraction
Event extraction is a vital component of Closenet, enabling it to identify and extract events and their attributes from unstructured text. This capability plays a crucial role in various NLP tasks and real-world applications.
- Identifying Events: Closenet can identify various types of events, including meetings, appointments, and financial transactions. It also recognizes the participants involved in the event, the time and location of the event, and other relevant attributes.
- Real-World Applications: Event extraction finds applications in multiple domains. In the healthcare domain, it can be used to extract events from medical records, such as patient appointments, surgeries, and medication administration. In the financial domain, it can be used to extract events from financial news and reports, such as mergers and acquisitions, earnings announcements, and stock market movements.
- Enhancing NLP Applications: Event extraction enhances the performance of NLP applications. In question answering systems, it enables the system to provide more comprehensive and informative answers by understanding the events and their attributes mentioned in the text.
- Facilitating Data Analysis: Event extraction also plays a vital role in data analysis tasks. By identifying events and their attributes in unstructured text, Closenet enables the extraction of structured data, which can be further analyzed to derive insights and make informed decisions.
In conclusion, Closenet's event extraction capabilities provide a deeper understanding of the events and their attributes mentioned in the text. This capability enhances the performance of NLP applications, facilitates data analysis tasks, and finds applications in various domains, making Closenet an essential tool for information extraction and text analysis.
Frequently Asked Questions about Closenet
This section addresses common questions and misconceptions surrounding Closenet, providing concise and informative answers to enhance understanding of its capabilities and applications.
Question 1: What are the key benefits of using Closenet?Closenet offers several key benefits, including enhanced information extraction accuracy, adaptability to new domains and languages, automated feature extraction and model building, scalability to handle large volumes of text data, and the ability to understand the context and meaning of text.
Question 2: How does Closenet's machine learning foundation contribute to its effectiveness?Closenet's machine learning foundation enables it to continually improve its accuracy, adapt to new domains and languages, automate feature extraction and model building, and handle large volumes of text data efficiently.
Question 3: What types of information can Closenet extract from text?Closenet can extract various types of information from text, including named entities (such as people, organizations, locations, and temporal expressions), relationships between entities, and events and their attributes (including participants, time, and location).
Question 4: How is Closenet used in real-world applications?Closenet finds applications in various domains, including question answering systems, text summarization, information retrieval, text mining, machine translation, legal document analysis, medical record analysis, and financial news analysis.
Question 5: What are the limitations of Closenet?Closenet, like any NLP tool, has limitations. It may encounter challenges in handling highly ambiguous or complex text, and its accuracy can be affected by the quality and diversity of the training data used.
Question 6: What are the future directions for Closenet's development?Ongoing research and development efforts focus on enhancing Closenet's accuracy, expanding its capabilities to handle new types of information extraction tasks, and improving its efficiency and scalability.
By addressing these frequently asked questions, we aim to provide a comprehensive understanding of Closenet's capabilities and applications, highlighting its significance in the field of natural language processing.
Transitioning to the next section of the article, we will delve deeper into the technical details of Closenet's architecture and algorithms, providing insights into its inner workings.
Conclusion
In conclusion, Closenet has emerged as a powerful tool in the field of natural language processing, revolutionizing the way we extract information from unstructured text. Its ability to identify and classify entities, relationships, and events, coupled with its machine learning foundation and natural language understanding capabilities, makes it an invaluable asset for a wide range of NLP tasks.
As we continue to explore the frontiers of NLP, Closenet is poised to play an increasingly vital role. Its ongoing development and the expansion of its capabilities hold immense promise for the future of information extraction and text analysis. By harnessing the power of Closenet, researchers, practitioners, and organizations can unlock the full potential of unstructured text data, leading to groundbreaking advancements in various fields.
Coway 2020 Year-End Promotion: Free Service For Faris Coway
Easy And Accurate French-English Translation With Linguee
Discover The Ultimate Puzzle Garage: Unravel Complex Puzzles With Ease

Secure Messenger & Proximity Sharing by OrangeLoops

Network Closet Clean Up 21st Century Network Cabling
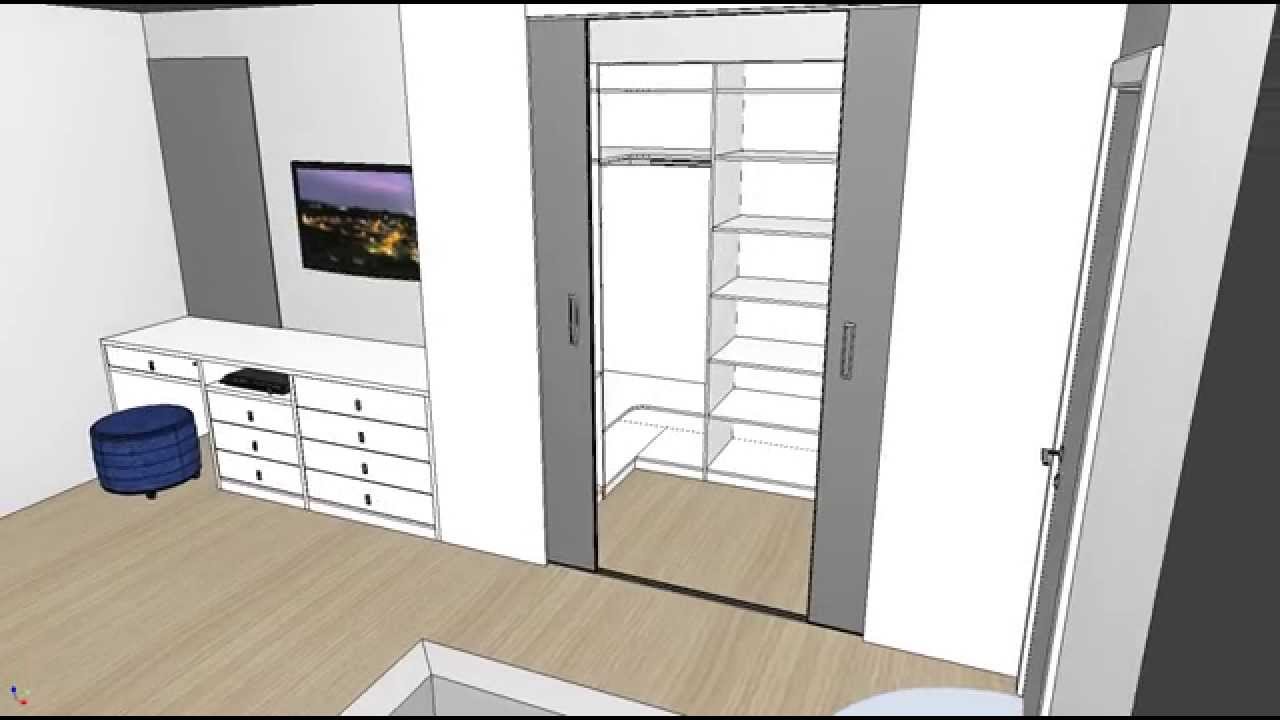
closett YouTube