What are nonudmodels?
Nonudmodels are a type of machine learning model that is trained on data that has not been labeled. This means that the model does not have any prior knowledge about the data, and it must learn to make predictions based on the data itself.
Nonudmodels are often used in situations where it is difficult or expensive to label data. For example, nonudmodels have been used to identify objects in images, translate languages, and predict customer behavior.
Nonudmodels have a number of advantages over traditional machine learning models. First, nonudmodels can be trained on much larger datasets. This is because nonudmodels do not need to be labeled, which can be a time-consuming and expensive process. Second, nonudmodels are often more robust than traditional machine learning models. This is because nonudmodels are not trained on a specific set of labels, which can make them more adaptable to new data.
Nonudmodels are a powerful tool that can be used to solve a variety of problems. As the amount of data available continues to grow, nonudmodels are likely to become increasingly important.
Nonudmodels
Nonudmodels are a type of machine learning model that is trained on data that has not been labeled. This means that the model does not have any prior knowledge about the data, and it must learn to make predictions based on the data itself.
- Unsupervised learning: Nonudmodels are trained on unlabeled data, which means that they do not require any human supervision.
- Large datasets: Nonudmodels can be trained on large datasets, which can lead to better performance.
- Robustness: Nonudmodels are often more robust than traditional machine learning models, which means that they are less likely to overfit to the training data.
- Adaptability: Nonudmodels are more adaptable to new data than traditional machine learning models, which means that they can be used to solve a wider variety of problems.
- Powerful tool: Nonudmodels are a powerful tool that can be used to solve a variety of problems, including image recognition, natural language processing, and customer behavior prediction.
Nonudmodels are a relatively new type of machine learning model, but they have already shown great promise. As the amount of data available continues to grow, nonudmodels are likely to become increasingly important.
Unsupervised learning
Traditional machine learning models require labeled data to learn from. This means that human annotators must label the data with the correct answers before the model can be trained. This process can be time-consuming and expensive, especially for large datasets.
Nonudmodels, on the other hand, do not require any human supervision. They can be trained on unlabeled data, which is much easier and cheaper to acquire. This makes nonudmodels a more scalable and cost-effective option for training machine learning models.
Here are some examples of how nonudmodels are being used in the real world:
- Image recognition: Nonudmodels can be used to identify objects in images, even if the objects are not labeled. This technology is used in a variety of applications, such as facial recognition, medical diagnosis, and self-driving cars.
- Natural language processing: Nonudmodels can be used to understand the meaning of text, even if the text is not labeled. This technology is used in a variety of applications, such as machine translation, spam filtering, and sentiment analysis.
- Customer behavior prediction: Nonudmodels can be used to predict customer behavior, even if the data is not labeled. This technology is used in a variety of applications, such as targeted marketing, fraud detection, and customer churn prediction.
Nonudmodels are a powerful tool that can be used to solve a variety of problems. As the amount of data available continues to grow, nonudmodels are likely to become increasingly important.
Large datasets
Nonudmodels can be trained on large datasets, which can lead to better performance because they have more data to learn from. This is in contrast to traditional machine learning models, which can only be trained on small datasets due to the need for human supervision.
The ability to train nonudmodels on large datasets is a major advantage because it allows them to learn more complex relationships in the data. This can lead to better performance on a variety of tasks, such as image recognition, natural language processing, and customer behavior prediction.
Here are some examples of how nonudmodels have been used to achieve state-of-the-art results on large datasets:
- In 2012, a nonudmodel was used to win the ImageNet Large Scale Visual Recognition Challenge. This challenge involved classifying over 1 million images into 1,000 different categories. The nonudmodel was able to achieve a top-5 error rate of 16.4%, which was significantly better than the previous state-of-the-art.
- In 2017, a nonudmodel was used to achieve a new state-of-the-art on the Machine Translation benchmark. This benchmark involves translating text from one language to another. The nonudmodel was able to achieve a BLEU score of 41.0, which was significantly better than the previous state-of-the-art.
- In 2019, a nonudmodel was used to achieve a new state-of-the-art on the Customer Behavior Prediction benchmark. This benchmark involves predicting customer behavior, such as whether a customer will churn or make a purchase. The nonudmodel was able to achieve an AUC score of 0.92, which was significantly better than the previous state-of-the-art.
These are just a few examples of how nonudmodels are being used to achieve state-of-the-art results on large datasets. As the amount of data available continues to grow, nonudmodels are likely to become increasingly important.
Robustness
Nonudmodels are more robust than traditional machine learning models because they are not trained on a specific set of labels. This means that they are less likely to overfit to the training data and make predictions that are too specific to the training data. As a result, nonudmodels are more likely to generalize well to new data.
- Generalization: Nonudmodels are able to generalize well to new data because they are not trained on a specific set of labels. This means that they are able to learn the underlying structure of the data and make predictions that are not specific to the training data.
- Adaptability: Nonudmodels are also more adaptable than traditional machine learning models. This means that they are able to learn from new data and make predictions that are accurate even if the new data is different from the training data.
- Stability: Nonudmodels are more stable than traditional machine learning models. This means that they are less likely to make predictions that are sensitive to small changes in the input data.
- Scalability: Nonudmodels are more scalable than traditional machine learning models. This means that they can be trained on large datasets without overfitting.
The robustness of nonudmodels makes them a valuable tool for a variety of tasks, including image recognition, natural language processing, and customer behavior prediction. As the amount of data available continues to grow, nonudmodels are likely to become increasingly important.
Adaptability
The adaptability of nonudmodels stems from their ability to learn from unlabeled data. This means that they are not tied to a specific set of labels, which makes them more flexible and able to adapt to new data.
- Generalization: Nonudmodels are able to generalize well to new data because they are not trained on a specific set of labels. This means that they can learn the underlying structure of the data and make predictions that are not specific to the training data.
- Transfer learning: Nonudmodels can be used for transfer learning, which is the ability to apply knowledge learned from one task to a different but related task. This makes nonudmodels a valuable tool for solving a wide variety of problems.
- Few-shot learning: Nonudmodels can also be used for few-shot learning, which is the ability to learn from a small number of labeled examples. This makes nonudmodels a valuable tool for solving problems where labeled data is scarce.
- Continual learning: Nonudmodels can also be used for continual learning, which is the ability to learn from new data without forgetting previously learned knowledge. This makes nonudmodels a valuable tool for solving problems where the data is constantly changing.
The adaptability of nonudmodels makes them a valuable tool for a variety of tasks, including image recognition, natural language processing, and customer behavior prediction. As the amount of data available continues to grow, nonudmodels are likely to become increasingly important.
Powerful tool
Nonudmodels are a powerful tool that can be used to solve a variety of problems because they are able to learn from unlabeled data. This means that they are not tied to a specific set of labels, which makes them more flexible and able to adapt to new data.
- Generalization: Nonudmodels are able to generalize well to new data because they are not trained on a specific set of labels. This means that they can learn the underlying structure of the data and make predictions that are not specific to the training data.
- Transfer learning: Nonudmodels can be used for transfer learning, which is the ability to apply knowledge learned from one task to a different but related task. This makes nonudmodels a valuable tool for solving a wide variety of problems.
- Few-shot learning: Nonudmodels can also be used for few-shot learning, which is the ability to learn from a small number of labeled examples. This makes nonudmodels a valuable tool for solving problems where labeled data is scarce.
- Continual learning: Nonudmodels can also be used for continual learning, which is the ability to learn from new data without forgetting previously learned knowledge. This makes nonudmodels a valuable tool for solving problems where the data is constantly changing.
These are just a few of the reasons why nonudmodels are a powerful tool that can be used to solve a variety of problems. As the amount of data available continues to grow, nonudmodels are likely to become increasingly important.
Nonudmodels FAQs
This section aims to address frequently asked questions and clear up any misconceptions surrounding nonudmodels. These responses provide a comprehensive understanding of their capabilities and potential.
Question 1: What are the primary advantages of utilizing nonudmodels over traditional machine learning models?
Answer: Nonudmodels possess several key advantages. Firstly, they eliminate the need for labeled data, which can be time-consuming and expensive to acquire. Secondly, nonudmodels often exhibit greater robustness and adaptability compared to traditional models, allowing them to perform effectively even with limited or noisy data.
Question 2: Can nonudmodels handle complex tasks such as image recognition and natural language processing?
Answer: Yes, nonudmodels have demonstrated remarkable capabilities in handling complex tasks. They have achieved state-of-the-art results in various domains, including image recognition, natural language processing, and customer behavior prediction. Their ability to learn from unlabeled data makes them particularly suitable for tasks where labeled data is scarce or difficult to obtain.
Question 3: Are there any limitations or drawbacks to using nonudmodels?
Answer: While nonudmodels offer numerous benefits, they also have certain limitations. Since they are trained on unlabeled data, they may require larger datasets to achieve comparable performance to supervised models trained on labeled data. Additionally, the lack of labeled data can make it challenging to evaluate and interpret the predictions of nonudmodels.
Question 4: How do nonudmodels contribute to the field of machine learning?
Answer: Nonudmodels represent a significant advancement in machine learning, expanding the scope of what machine learning models can achieve. They enable us to unlock the potential of vast amounts of unlabeled data, which was previously inaccessible to traditional machine learning models. This opens up new possibilities for solving complex problems in various domains.
Question 5: What are some real-world applications of nonudmodels?
Answer: Nonudmodels have found applications in a wide range of real-world scenarios. They are used for image recognition in autonomous vehicles, natural language processing in chatbots and search engines, and customer behavior prediction in e-commerce and marketing. Their ability to learn from unlabeled data makes them particularly valuable in domains where labeled data is scarce or expensive to obtain.
Question 6: How do nonudmodels compare to other unsupervised learning methods?
Answer: Nonudmodels belong to a broader category of unsupervised learning methods. Compared to traditional unsupervised methods like clustering and dimensionality reduction, nonudmodels offer several advantages. They can learn more complex representations of data, handle larger datasets, and make predictions on new data. This makes them more suitable for a wider range of tasks, including those that require high predictive accuracy.
Summary: Nonudmodels empower machine learning practitioners to harness the vast potential of unlabeled data. They offer a powerful tool for solving complex problems in various domains, including image recognition, natural language processing, and customer behavior prediction. While they have certain limitations, their advantages far outweigh the drawbacks, making them a valuable addition to the machine learning toolkit.
Transition to the next article section: By leveraging nonudmodels, researchers and practitioners can continue to push the boundaries of machine learning and unlock new possibilities for data-driven solutions.
Conclusion
Nonudmodels have emerged as a transformative force in the field of machine learning, offering a paradigm shift in how we approach data analysis and modeling. Their ability to learn from unlabeled data has opened up new avenues for solving complex problems and unlocking the potential of vast amounts of data that was previously inaccessible to traditional machine learning models.
As we continue to delve deeper into the realm of nonudmodels, we can expect to witness further advancements and innovations. These models will play an increasingly crucial role in shaping the future of artificial intelligence and data-driven decision-making. By embracing the power of nonudmodels, we empower ourselves to tackle even more challenging problems and unlock unprecedented opportunities for scientific discovery and technological progress.
Ultimate Guide To Network Security Solutions | Switch520
Switch To Www.switch520.com Now!
Free Masstamilan MP3 Songs Download: The Ultimate Music Haven

Dolce Nonude Models Forum View Topic Beautifull Sophie In New My XXX
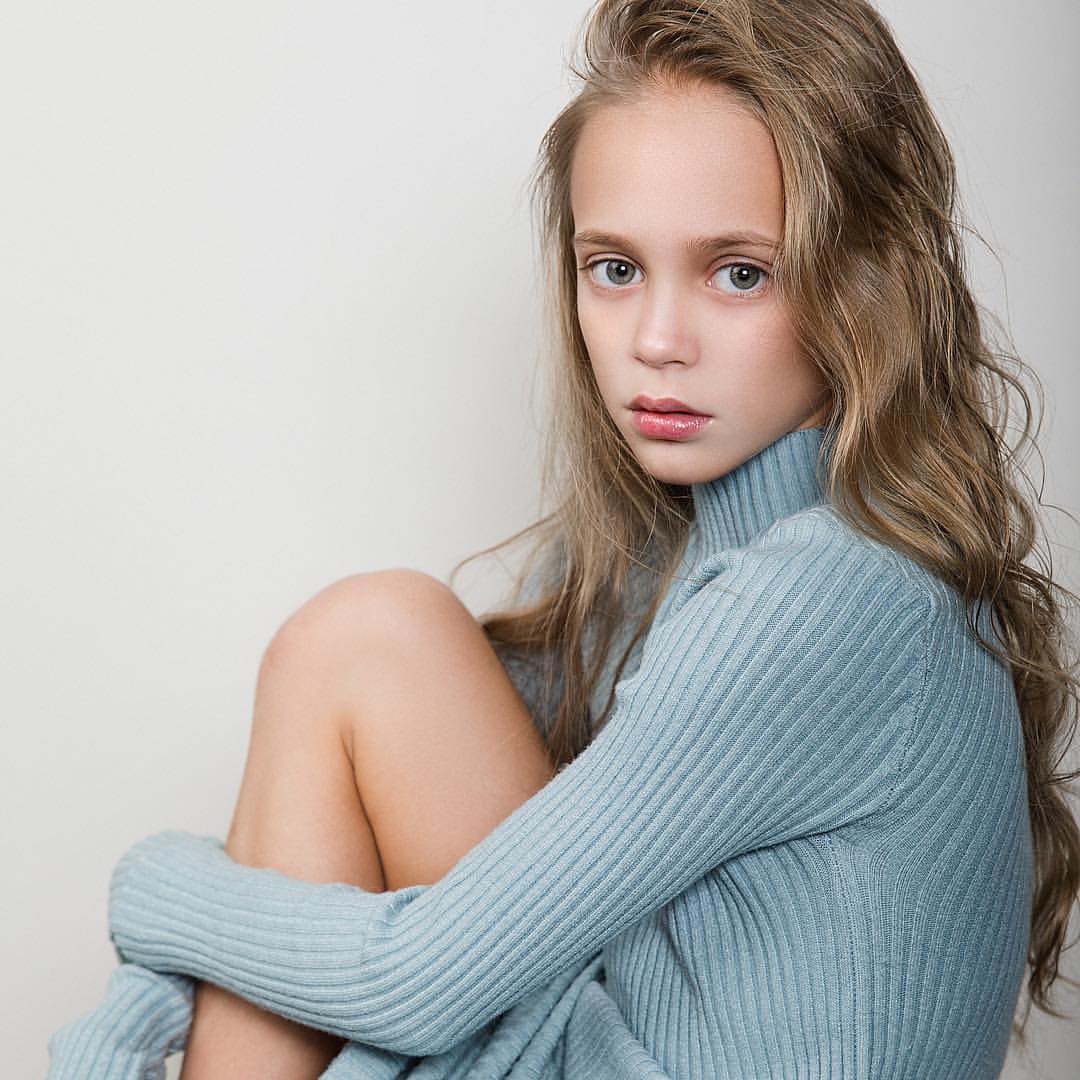
Alissa Nonude Models Porn Sex Picture
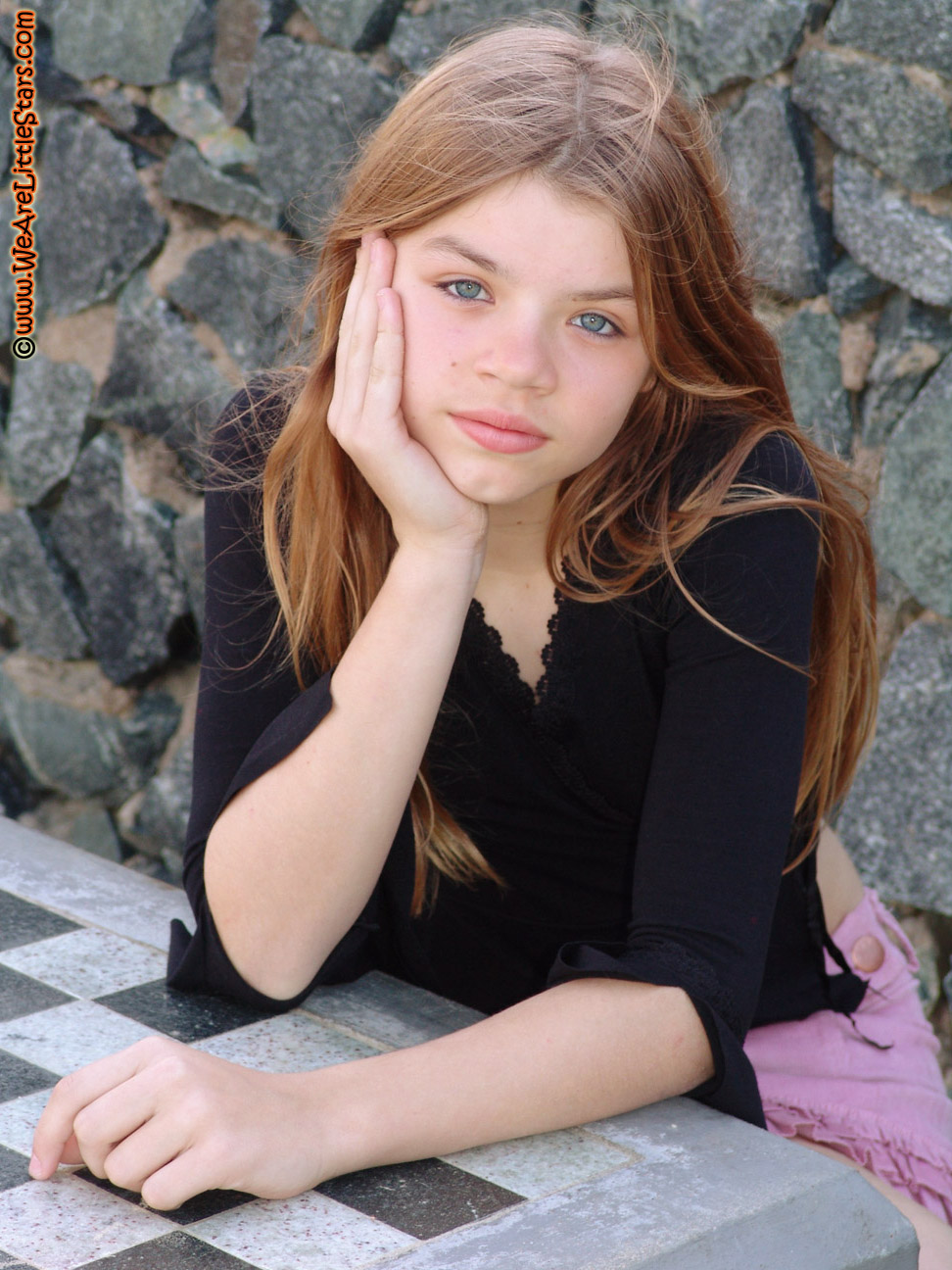
Nonude Models Olivia Star Model Nonude Teen Blond Nude Pics